Lehmer Transform for Complex Data Analysis
About
We discuss the Lehmer Transform which has been recently proposed to compress and characterize large volumes of highly volatile and nonstationary time series data like financial and biological signals. Subsequently, all complex signals can be analyzed under one coherent and unified framework capable of concentrating on patterns that are difficult to capture by classical methods such as Wavelets or Fourier Transforms. The model has many other advantages such as: 1- it is easily interpretable; 2- there is no need for preprocessing 3- there is no need for noise removal; 4- there is no need to store large volumes of data since all calculations can be carried out online; 5- it can be used for solving both supervised and unsupervised machine learning problems.
Speaker
Masoud Ataei is currently a professor of mathematics at the University of Toronto and a lead AI scientist at the University of York.
​
Hansen Chen is currently a theoretical physics Ph.D. at Yale.
​
Abyss is a Data scientist/machine learning engineer, biomedical and chemical engineer, with published work in stochastic rheology and reaction modeling, parametric finite element human body modeling, cerebral autoregulation signal processing, and machine learning for brain implants in a Parkinson study.
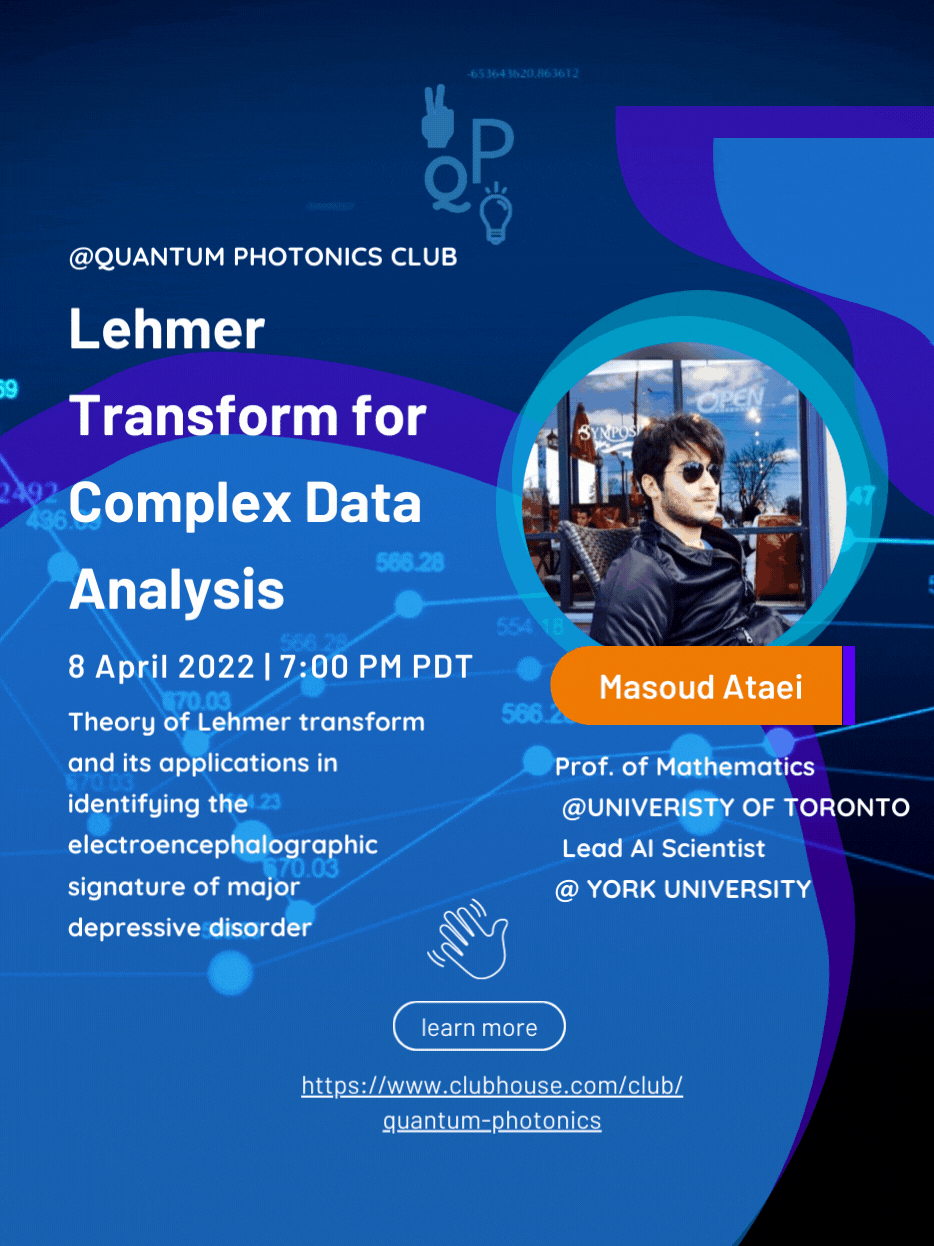